Like the advent of other truly transformative technologies like personal computers, the internet, or content streaming services, artificial intelligence (AI) is on a similar fast-track toward mainstream business use, including in the financial services industry. While every financial institution is on its own journey of adopting AI in their various business functions, there are certain key benefits of using machine learning, a type of AI, for decisioning, especially in assessing credit risk, that compel those institutions to revisit their lending models.
Benefit #1: Faster processing
While traditional lending models offer some benefits (e.g., relying on existing internal expertise and the explainability of the models), one area that remains a challenge for financial institutions is the time it takes to build and develop credit risk models, which can take months. This duration jeopardizes business agility should world events or market conditions cause sudden shifts in consumer behavior.
Machine learning-driven advanced analytics and models can increase the accuracy and effectiveness of decisioning throughout a customer life cycle with lending, detecting fraud, managing collection efforts, and much more. These models can automate repetitive or manual internal tasks and enhance the customer experience with externally facing functions such as web-based chat, customer call centers, and credit/financial education tools.
Benefit #2: Democratization of AI
Tools like ChatGPT and other generative AI front-end interfaces are doing for machine learning and AI what web browsers like Netscape and Internet Explorer did for the internet in the 1990s. They provide a means for far easier use and greater accessibility to the technology and remove it from being only in the purview of data scientists and engineers.
This democratization with generative AI enables everyone to harness and innovate through the power of AI. Forward-looking companies see this opportunity and are proliferating the technology among their employees in responsible ways to spark internal grassroots innovation, accelerate product and service development and go-to-market strategies, and expand financial inclusion in their offerings.
Benefit #3: Better Data Utilization
When it comes to decisioning, machine learning approaches to analytics and modeling have existed for some time. However, advances in computer science and computing power further strengthened the speed and capabilities of machine learning models, especially with processing data at scale. Now, these models can quickly ingest an organizationâs internal data, alternative data, credit bureau data, credit attributes and other scores to provide a more accurate and holistic view of an applicationâs riskiness.
In this case, the model estimates the creditworthiness of an applicant so lenders can make better-informed decisions. As these models grow in sophistication and an increased amount of data becomes available, they can more accurately segment and score populations, which will result in fewer applications going to manual review, which can save money and improve the customersâ experiences.
Even more exciting, AI-enabling companies have developed sandbox platforms to empower business professionals and data scientists alike to experiment with models using anonymized, privacy-compliant data to try new approaches, research market trends and consumer interest, and mitigate risk factors before launching an offering.
Benefit #4: Data-empowered personalization and sentiment analysis
Like the retail ecommerce industry, financial institutions are building online presences that are easy to use and fast, and deliver personalized service based on a userâs digital identity, which is comprised of every-day digital interactions â email address, search queries, geotags, behaviors and device information â that help authenticate that user.
AI can take this personalization to another level by employing tools such as sentiment analysis (i.e., determining the emotional tone of communications as positive, negative, or neutral) to evaluate behaviors over time to offer relevant recommendations that ease consumer decisionâmaking and information input. This works much in the same way as how Netflix offers movie recommendations based on past viewing or Spotify does with music.
As financial institutions assess their portfolio, evaluate their risk categories, and determine areas of opportunity with different audience segments, they can tailor their machine learning models to make the right offers to the right consumers at the right time to optimize the likelihood of them accepting.
Benefit #5: Promote Explainability
The issue of explainability for machine learning models is critical. Defining the term, the output of a model is explainable when its internal behavior can be directly understood by humans or when explanations can be provided for the main factors that led to its output. One of the primary concerns that financial institutions have about machine learning models involves âblack boxâ models, where the institutions cannot verify how the models work internally or explain why decisions are made to regulators or consumers.
According to recent research conducted by Forrester Consulting, lack of explainability was the biggest barrier to machine learning adoption by businesses. Financial institutions should ensure that their models are easily interpretable by humans who evaluate the output and can generate reason codes that help consumers understand outcomes that occur.
Developers can use techniques, such as gradient boosting machines (GBMs), which is a type of highly predictive, transparent machine learning algorithm, to create more efficient models that are explainable and do not disproportionately affect protected classes. For example, financial institutions can understand why an applicant was rejected for credit, along with the key drivers behind their low credit score, such as low-income ranking. This information can be translated into reason codes and used for regulatory and auditing purposes.
Conclusion
Machine learning models for AI-driven decisioning can help financial institutions increase financial inclusion, mitigate risk, dramatically improve the customer experience, initiate more loans, produce regulatorily compliant reports, grow employee engagement, and a host of other favorable outcomes, all while reducing overall costs. If a company doesnât have the internal bandwidth to fully pursue implementing AI in their operations or lacks domain knowledge, they should work with a partner that is well-versed in machine learning approaches and AI to help navigate how to leverage this vitally important technology.
Stay Ahead of the Financial Curve with Our Latest Fintech News Updates!
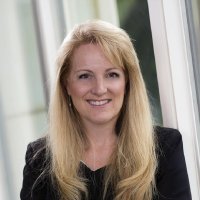
Kathleen Peters, CIO at Experian Decision Analytics
Kathleen Peters leads innovation and business strategy for Decision Analytics in North America in her current role as Chief Innovation Officer. She and her team continuously look for new ways to define product strategies, road maps and priorities for Experianâs identity and fraud portfolio, and analyze industry trends and the latest technologies to bring innovative solutions to the companyâs clients.
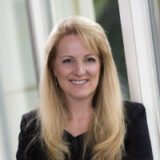