Credit risk modeling is a method for lenders to understand how convincing a particular loan is to get repaid. Especially, it is a tool to understand the credit risk of a borrower. This is particularly important because this credit risk profile continues to change with time and circumstances.
Over the last decade, a number of the largest banks all around the world have built sophisticated systems in an attempt to model the credit risk arising from important aspects of their business lines. Such models are intended to help banks in aggregating, measuring, and managing risk across product lines and geographies. The yields of these models also play significant roles in risk management and performance measurement processes of banks, incorporating performance-based compensation, risk-based pricing, customer profitability analysis, and, to a lesser but growing degree, capital structure decisions, and active portfolio management.
What is credit risk?
Credit risk indicates the chance that a borrower will fail to make their payments on time and default on their debt. It refers to the risk that a lender may not receive the principal lent or their interest due on time.
This results in the interruption of cash flows for the lender and increases the cost of collection. In severe cases, some parts of the loan or even the entire amount lent may have to be written off resulting in a loss for the lender.
It is extremely difficult and also very complex to pinpoint exactly how possibly a person is to default on their loan. At the same time, properly assessing credit risk can decrease the probability of losses from default and delayed repayments.
Interest payments from the borrower are the reward of the lender for bearing credit risk. If the credit risk is higher, the lender or investor will either change a higher interest or go without the lending opportunity altogether. This means a loan applicant with a good credit history and steady income will be charged a lower interest rate for the same loan when compared to an applicant with poor credit history.
What is credit risk modeling?
There are numerous different factors that affect the credit risk of a person. This makes accessing a credit risk of a borrower a highly complex task. With so much money riding on the ability to accurately estimate the credit risk of a borrower, credit risk modeling has come into the picture.
Credit risk modeling refers to the process of utilizing data models to find out two important things, first is the probability of the borrower defaulting on the loan and second is the impact on the financials of the lender if this default occurs.
Financial institutions depend upon credit risk models to determine the credit risk of potential borrowers. They make decisions on whether or not to sanction a loan as well as on the interest rate of the loan based on the validation of the credit risk model.
As technology has progressed, new ways of modeling credit risk have emerged including credit risk modeling using R and Python. These include utilizing the latest analytics and big data tools to model credit risk. Other factors like the evolution of economies and the subsequent emergence of different types of credit risk have also affected how credit risk modeling is done.
Types of credit risk
There are various different types of credit risk which emerge based on the type of loan and situation. Apparently, different credit risk models work better for different kinds of credit and credit risk model validation differs accordingly. Here we have listed some common credit risks that lenders undertake.
- There is a risk that an individual borrower may fail to make a payment due, on various credits/loans.
- A business or individual fails to pay a trade invoice on the due date. This is a common risk that both B2C and B2B businesses that work on credit carry.
- An organization that borrows money is unable to repay fixed or floating charge debt.
- An insurance company that is insolvent does not make a claim payment which is due.
- A government or a company may have issued a bond that it does not pay the interest or principal amount on.
- A business does not pay an employee’s wages or salary when they become due.
- A bank that is now bankrupt doesn’t return money that has been deposited.
Factors affecting credit risk modeling
The risk for the lender is of various kinds ranging from disruption to cash flows, and increased collection costs to loss of interest and principal. That is why it’s important to be able to forecast credit risk as precisely as possible. Credit risk modeling depends on several complex factors. That’s why it is important to have sophisticated credit risk rating models.
These are several factors to consider while determining credit risk. From the financial health of the borrower and the consequences of a default for both the debtor and the creditor to a variety of macroeconomic considerations. Here are the major factors affecting the credit risk of a borrower.
- The probability of default (PD)
This invokes the likelihood that a borrower will default on their loans and is obviously the most important part of a credit risk model. For a person, this score is based on their debt-income ratio and existing credit score.
For institutions/companies that issue bonds, this probability is determined by rating agencies like Moody’s and Standard &Poor’s. The PD normally determines the interest rates and amount of down payment required.
- Loss Given Default
This indicates the total loss that the lender will suffer if the debt is not repaid. This is a vital component in credit risk modeling. For example, consider two borrowers with the same credit score and a similar debt-income ratio will present two different credit risk profiles if one is lending a much larger amount.
That is because the loss to the lender in case of default is much higher when the amount is larger. This again plays a key role in determining down payments and interest rates. If the borrower is willing to offer collateral, then that has a big impact on the interest rate offered.
- Exposure to Default
This is a measure of the total exposure that a lender is exposed to at any given point of time. This also has an impact on the credit risk since it is an indicator of the risk appetite of the lender. It is calculated by multiplying each loan by a specific percentage depending upon the particulars of the loan.
Types of credit risk rating models
Credit risk modeling relies on how effectively you can leverage data about the financial history, income, and so on about a borrower, to arrive at an accurate credit score. Analytics and Big data are allowing credit risk modeling to become more scientific as it is now based more on past data than guesswork. In fact, credit risk modeling using R, Python, and other programming languages is turning out to be more mainstream. Here’s an excellent video that discusses different credit risk, rating models.
Obviously, the ultimate credit risk model validation comes only after there are years of data to back the accuracy of a forecast.
Here are the three major types of credit risk rating models that are utilized to determine credit risk.
- Based on Financial Statement Analysis
Examples of these models involve the Altman Z score and Moody’s Risk Calc. These models are based upon an analysis of the financial statements of borrowing institutions. They essentially take into account well-known financial ratios that can be useful in determining credit risk. For a moment, the Altman Z score takes into account financial ratios like total EBIDTA/ taxes and sales/total assets in various proportions to decide the likelihood of a company going bankrupt.
- Measuring Default Probability
The perfect example of this kind of credit risk modeling is structural models like the Merton model. Structural models consider business failures to be an endogenous occasion that relies upon the capital structure of the organization. In other words, they operate on the assumption that a business will fail and default on its loans if its value falls below a specific threshold.
- Machine learning Models
The initiation of machine learning and big data to credit risk modeling has made it possible to develop credit risk models that are more scientific and accurate. An extraordinary example of this is the Maximum Expected Utility model which is based on machine learning.
While the MEU model was introduced as early as 2003, it has now integrated several elements of machine learning to predict credit risk more accurately. In fact, many credit risk calculations including the famous FICO score are currently adding scores from machine learning models to score from traditional models to improve accuracy.
Conclusion
There are still various approaches to credit risk modeling and different approaches work better in different scenarios of lending. Of course, credit risk modeling has additionally become more advanced, particularly with newer analytics tools.
Credit risk modeling utilizing R, Python, and other analytics-friendly programming languages have significantly improved the ease and accuracy of credit risk modeling. Credit risk modeling is still intensely niche and offers great career prospects for those who have a good grasp of analytics as well as the world of finance.
We hope that you have got a clear picture of how predictive modeling is utilized in the credit risk domain and what are the key credit risk parameters. In risk analytics, knowledge of the domain is more important than technical or statistical knowledge.
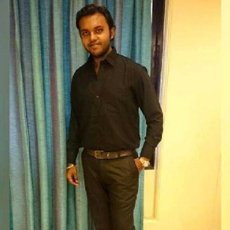
Aashish Yadav, Content-Editor, FintecBuzz
Aashish is currently a Content writer at FintecBuzz. He is an enthusiastic and avid writer. His key region of interests include covering different aspects of technology and mixing them up with layman ideologies to pan out an interesting take. His main area of interests range from medical journals to marketing arena.