The task of financial crime compliance has become an increasingly complex endeavor for major financial institutions worldwide. As the magnitude and repercussions of global money laundering escalate, these institutions are grappling with a complex web of risk and regulation. This intricate landscape has sparked a phenomenon known as ‘de-risking’, born out of a need for self-preservation but carrying unintended consequences.
De-risking is the practice where financial institutions terminate or restrict business relationships with entire categories of clients to reduce their exposure to potential financial crime. This is not a nuanced approach based on detailed risk analysis of individual clients. Instead, it’s a sweeping, indiscriminate policy that while reducing immediate risk, is also causing significant collateral damage.
The US Department of the Treasury has voiced its concerns:
Correspondent Banking
Correspondent banking, a crucial cog in the machinery of global finance, has been hit particularly hard by de-risking policies. In a correspondent banking relationship, a larger financial institution (the correspondent bank) provides services on behalf of a smaller or equally sized institution (the respondent bank). These services can include wire transfers, business transactions, trade finance facilitation, and document collection.
The Financial Conduct Authority (FCA) in the UK noted in 2016 that “some banks are withdrawing from providing correspondent banking services.” This observation was substantiated by a 2019 report from the Bank of International Settlements (BIS), which highlighted a concerning 22% drop in the number of active correspondent banks globally from 2011 to 2019.
Corresponding banking, by its nature, carries significant risk. When correspondent banks provide services to the clients of respondent banks, they take on the liability for those clients’ behaviors and actions. This liability is a considerable concern for the many financial institutions looking to minimize their exposure to financial crime, and some have turned to de-risking policies. The goal of such policies is to reduce potential risk, but the consequence is a broad, sweeping withdrawal from correspondent banking relationships, impacting not only these institutions, but the individuals and businesses that benefit from these services, and the global financial system at large.
The fallout of such de-risking strategies is felt most acutely by respondent banks in developing nations. These nations, often perceived as having weaker governance and higher incentives for financial crime, bear the brunt of these policies. As a result, respondent banks in developing nations find themselves disproportionately excluded from international financial services.
- A 2017 survey by the Caribbean Association of Banks found that 21 of the 23 banks in 12 Caribbean countries had lost at least one correspondent banking relationship.
- Research published by The World Bank in November 2015, Withdrawal from Correspondent Banking, found that over half of the banks surveyed reported a moderate or significant decline in their correspondent banking relationships in Africa.
- Angola experienced a loss of more than 37% of its foreign counterparties between 2013 and 2015.
These damaging outcomes highlight the need for solutions that enable a more measured and nuanced approach to risk management.
Federated Learning: Enabling Nuance, Unlocking Value
What is Federated Learning?
Federated Learning is a method that allows machine learning models to be trained across multiple decentralized devices or servers. The crucial distinction here is that instead of mobilizing data, Federated Learning mobilizes models. This offers a significant advantage, especially when dealing with sensitive information.
Data, by its very nature, is explicit and therefore potentially sensitive. On the other hand, models aim to capture the patterns and trends seen in data. They distill the insights while leaving the explicit, sensitive details behind. In the Federated Learning approach, data stays local, residing in its original location. This eliminates the many restrictions and concerns associated with data sharing and privacy. Instead of moving data, the model itself is deployed and trained across various firms’ local environments.
Each participating entity independently trains a version of the model using its local data. These separately trained models are then aggregated to form a single ‘champion’ model. This central model encapsulates the learnings from each local environment, offering a nuanced understanding that a single, centrally-trained model could not achieve. In this way, Federated Learning is not just a technical innovation, but a pathway towards unlocking new value in machine learning, fostering both data privacy and global insights.
How does Federated Learning Combat De-Risking?
Federated Learning, by enabling the deployment of technology at correspondent banks in high-risk jurisdictions, presents a unique opportunity to tackle the complications of de-risking. It does so by enhancing control, assurance, and accuracy, thereby addressing several key challenges in this area.
Federated Learning directly addresses the issue of control, a fundamental challenge in correspondent banking. The risk in this sector often stems from the correspondent bank’s limited oversight over the respondent’s clients. Federated Learning offers a solution to this dilemma. By sharing technology through this approach, banks can maintain a stronger grasp on the risk management tools used to assess clients in high-risk jurisdictions. This not only provides better control but also delivers enhanced assurance. With a direct involvement in the ‘champion’ model, correspondent firms can scrutinize, test, and request changes to these models as necessary, ensuring they accurately reflect the institution’s risk tolerance and regulatory requirements. This combined control and assurance foster a more confident and secure approach to managing risk in correspondent banking relationships.
The inherent strength of Federated Learning lies in its accuracy and robustness. The ‘champion’ model, trained on heterogeneous datasets across different entities, has the opportunity to encode a wide spectrum of risks. This broad exposure enables the creation of models that are not only more accurate in detecting financial crime but also more robust to the inevitable variations across different jurisdictions, customer types, and behaviors implicated in correspondent banking.
Ultimately, the assurance derived from these more accurate and controllable models can inspire larger banks to adopt a more discerning, case-by-case approach to risk management, replacing broad de-risking strategies with targeted, data-driven decisions.
What are the Benefits?
By reversing the trends set by historical and prevailing de-risking strategies, Federated Learning brings about significant and wide-reaching benefits for a diverse range of stakeholders:
- Revenue and Growth for Correspondent Banks: Federated Learning opens up new avenues for business and growth, enabling correspondent banks to engage confidently with high-risk jurisdictions. This directly translates into diversified portfolios and increased revenue streams.
- Increased Financial Inclusion: The approach promotes economic growth and fosters social mobility by supporting legitimate business operations, especially in small jurisdictions. This reduces the long-term incentives for financial crime and aids sustainable growth in sectors like tourism and trade that are crucial to many smaller economies.
- Integrity of the Global Financial System: By combatting de-risking, Federated Learning helps to maintain transparency and protect the integrity of the global financial system. It prevents individuals and businesses from resorting to unregulated financial channels, thereby reducing the risk of sanctions evasion and the creation of profit centers for criminals.
Federated Learning offers a new paradigm in which banks can reduce financial crime risk exposure without resorting to heavy-handed policies. This approach opens up revenue and growth opportunities while fostering global financial inclusion, benefiting individuals, businesses, and communities.
[1] https://www.fca.org.uk/firms/money-laundering/derisking-managing-risk
[2] https://www.bis.org/cpmi/paysysinfo/corr_bank_data/corr_bank_data_commentary_2008.htm
[3] https://www.worldbank.org/en/news/feature/2018/05/02/are-global-banks-cutting-off-customers-in-developing-and-emerging-economies
[4] https://www.csis.org/analysis/there-new-normal-de-risking-caribbean
[5] https://www.oecd.org/corruption/illicit_financial_flows_from_developing_countries.pdf
[6] https://www.thebanker.com/De-risking-in-Africa
[7] https://www.swift.com/news-events/press-releases/de-risking-africa-rise-according-latest-swift-data
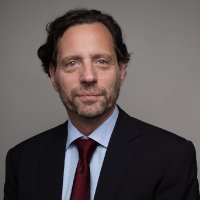
Laurence Hamilton, Chief Commercial Officer, Consilient
Laurence brings extensive global business leadership experience. Prior to Consilient, Laurence was Group Managing Director of a Data, Analytics and Software company, successfully growing the organization in Europe, North America and Australia. Most recently Laurence has been instrumental in the successful launch of a new UK digital bank overseeing all commercial aspects including branding, pricing, marketing and products. Laurence brings substantial P&L leadership, turnaround & growth expertise.
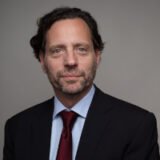
Laurence Hamilton
Laurence brings extensive global business leadership experience. Prior to Consilient, Laurence was Group Managing Director of a Data, Analytics and Software company, successfully growing the organization in Europe, North America and Australia. Most recently Laurence has been instrumental in the successful launch of a new UK digital bank overseeing all commercial aspects including branding, pricing, marketing and products. Laurence brings substantial P&L leadership, turnaround & growth expertise.