The insurance industry has recently seen tech-driven growth emerge in more traditional areas such as underwriting, claims management and fraud detection, and often as a direct result of new insurtech models being introduced into the market. Even so, insurance pricing has not evolved, being both a core and highly regulated process. While technological innovations such as Artificial Intelligence and Machine Learning are beginning to be leveraged by a number of pricing teams, this is mostly on an exploratory basis, with a test and learn approach that cannot be used in production or filed for regulatory purposes. In general, the pricing process has remained a ‘dark niche’, mastered by a few technical experts, often using manual legacy tools.
A Perfect Storm
Today’s market environment has drastically shifted due to multiple factors listed below, all of which have led to a perfect storm within the insurance industry and urgent need for rapid growth:
1. Covid 19
2. Growing competitive pressure from disruptors and GAFAs1
3. The rise of insurtechs
4. Evolving customer standards
5. Increasing demand for new value creation and differentiation levers (See our position paper “The Transformation Imperative for Insurers” for a deep dive on this topic.2)
For example, Covid 19 was an unprecedented accelerator of change for the insurance industry. To reference just one data point: Salesforce3 has predicted that the insurance market will contract due to an expected global GDP decrease of at least 5.2%. And coming out of the pandemic, insurers are more than likely to face even more challenging market conditions.
To stay afloat in this ‘New Deal’ era, insurers need to explore undisrupted areas within the insurance value chain to unlock new potential. Due to unique requirements within the insurance industry, pricing sophistication is one example of this new untapped frontier, and a very attractive one when well executed.
The risks of insurance pricing
Like in all sectors, pricing is at the heart of business decisions, but there are several factors that make the pricing process very specific to the insurance industry:
1. Unknown costs: When an insurer establishes the price of an insurance policy, they have little certainty regarding how much that policy will ultimately cost the company. Best case scenario, final costs will be determined three to four years later, after claims have occurred, with various levels of frequency and severity.
2. Adverse selection risks: Adverse selection for insurers occurs when an insurance company charges a policy subscriber lower premiums than their actual risk profile would call for. An insurer that underestimates a customer risk profile and as a result underprices that policy will attract not one, but potentially all the risky profiles in the market. Compared to other industries, this heavy share of high-risk profiles, along with the length of time that passes before they uncover this error and costs materialize, generate a disproportionate impact compared to the initial pricing error. And of course, insurers struggling with adverse selection are unintentionally helping their competitors become more profitable.
3. Regulatory constraints: Insurance pricing is heavily regulated, with the nature and depth of regulations differing by market. Requirements include filing obligations, retail margin control over technical prices, number and type of variables that can be used, and the list goes on and on. The level of scrutiny borne by insurers makes pricing a highly sensitive topic, and calls for utmost accountability and thus, transparency.
4. Distribution constraints: Intermediated insurers need their pricing strategy to be as transparent and explainable as possible to their agents, to maximize their willingness to adopt these strategies .
5. Repricing imperatives: Risk and demand-based pricing components are subject to change. While major phenomena, such as natural disasters or economic crises may significantly alter customers’ risk profiles, the demand component is structurally subject to more repeated and material modifications. Ongoing changes in behavioral patterns and competitive pricing call for an almost continuous review and adaptation of policy pricing.
6. Conflicting injunctions: Increasing portfolio performance standards imply the need for evermore sophistication in rate modeling parameters (i.e., more variables, integration of behavioral data, etc.) to optimize GWP and loss ratio. Conversely, user experience focused strategies require simple quoting and subscription processes to maximize conversion with a minimum of clicks, implying fewer questions asked to customers and therefore less information gathered.
The many challenges of a robust pricing strategy
Insurance pricing is both art and science.
Its specificities tend to make it a “dark niche”, mastered by a few chosen ones, notably actuaries, a sacred profession in the insurance industry. As a result, decision drivers that lead to rate computation can be unclear to the laymen.
Because the need for transparency is so enshrined in the rate making process, innovation has shied away from this space for many years. Ancient-looking, manual tools are the norm. Prices are commonly updated at best once a year, at a prudent pace with lengthy time to market. Eight months to update the price of a car insurance policy, or a year to launch a new product on the market are not uncommon data points. As a result of these conditions, we see insurance pricing as ripe for disruption.
Fortunately, the emergence of Machine Learning (ML) techniques like GBMs (Gradient Boosting Machines) or Random Forest paved the way for speed and performance gains. But it’s critical to note that applying these classic ML techniques to pricing have encountered limitations because of the blackbox nature of such algorithms. Blackbox ML can expose carriers to risks of adverse selection, with significant financial impact if ML is misused in pricing decisions. This is why these types of models are often used for exploratory purposes, and not in production, given the adverse selection and regulatory risks induced.
Delivering pricing sophistication is undeniably a complex challenge, though not impossible!
The next value reservoir for insurers
Two main strategies stand out for unlocking the value of pricing sophistication:
1. The ability to harness data (whether internal or external) to embrace data-driven pricing. This first one is becoming an industry norm. Data sources are multiplying. Telematics allow insurers to capture new data, with greater accuracy and granularity. Technology provides insurers with the ability to see not only how individuals drive their car, but also under what circumstances, i.e. traffic, road conditions, time, mood, etc. That combination of information is a more powerful predictor of insurance losses than pure demographic information such as age, gender, marital status, or where the car is garaged. Hence, the opportunity to get more granular in how prices are set is a win-win combination for both the carrier and the customer, reducing risks and losses.
2. Using ML powered algorithms in production. The key accelerator and success factor in pricing is moving from exploration in data labs to the production stage, to leverage the power of ML at scale and generate sizable business impact. This is where Transparent ML comes into play. Transparent ML-powered algorithms harness the power of ML while preserving complete control, auditability and transparency over the models created. Transparent ML uniquely combines actuarial and data sciences, generating models that are production-ready, based on standards that actuaries know and use: Generalized Linear Models (GLMs).
But wait, there’s more to successful pricing sophistication than that!
Remember how insurance pricing is both art and science? Well, algorithms take care of the science, and pricing teams perfect the art.
Indeed, the pricing sophistication journey calls for broader considerations:
1. Automating data-driven processes like rate modeling to gain speed-to-accuracy calls for the best-in-class automation tools, with built-in transparency and the ability to go into production.
2. It also calls for a renewed and augmented role of pricing teams, with less time spent on repetitive, manual modeling tasks and more focus on value-added business input.
3. The augmented role of pricing teams will empower them to gain business relevance and impact across the organization, leveraging the value and best practices of AI-based solutions.
Bottom line: what’s really in it for insurers and policyholders?
Embarking on the pricing sophistication journey is a win-win for insurers and end customers.
An insurer’s pricing sophistication journey gradually evolves from the use of GLMs for risk modeling, to building competition-based pricing capabilities – running “what if” scenarios – all thanks to best-in-class pricing automation tools used in production.
Insurers that progress along this journey will unlock GWP and loss ratio improvement potential, through performance, speed and reliability gains, increased predictive power and accelerated time-to-market. McKinsey4 has estimated the impact of the pricing sophistication journey on insurers’ loss ratios:
1. The first step, the consistent application of GLMs, yields up to 1.5pp for acquisition and 0.2 to 0.5pp for renewal
2. Full-scale pricing transformation can generate a whole 3 to 6pp in loss ratio improvement.
3. 3-4% additional GWP growth can be achieved through better acquisition and retention performance.
Sophisticated pricing solutions empower insurers to make the best-informed conscious business decisions, based on reliable and robust outputs.
Down the road, policyholders are most likely to benefit from higher personalization through more targeted and better-adjusted prices that account for their behaviors, usage patterns, competitive pricing and such factors. The level of understanding and precision brought by such solutions also means greater transparency by their insurer, a decisive factor to (re)build trust in an industry that suffers from a great lack of it.
Conclusion
No insurer would dispute the core importance of pricing within their strategy. Just like no insurer would argue the irreplaceable strategic value of pricing teams. Yet pricing teams are largely under-equipped, too often relying on ancient manual tools to work their magic.
Pricing sophistication can address this paradox, opening a crack into a major and vastly untapped value reservoir for insurers. This journey must come with the desire to embrace a renewed vision and understanding of the importance of pricing in the data & tech era. It also calls for adapted rate modeling tools leveraging AI with all insurance pricing constraints in mind. These will be game-changers, empowering the organization, with pricing teams sitting in the driver’s seat, allowing the power of ML to graduate from data labs to production status for maximum impact. As Munich Re noted, “These technological advancements are at the base of the Automated Machine Learning (AutoML) field which is changing the role of the pricing expert. AutoML commoditises the prediction, allowing the pricing actuary to focus on the decision-making process and the implementation.”5
In times of unprecedented uncertainty, sophisticated pricing teams will empower insurers to quickly react and adapt to changes and make the most of them.
That is if insurers want to stay in the game.
For more information, visit www.akur8.com or contact us at contact@akur8.com
1- GAFAs – the four largest, most dominant, and most prestigious tech companies in the information technology industry of the United States including Google, Amazon, Facebook and Apple
2- ˆThe Transformation Imperative for Insurers” https://assets.website-files.com/602146d5f44c88037ab480a0/602ab3cfea370270e1dfc4f1_The%20transformation%20imperative%20for%20insurers.pdf
3- Building the Bionic Insurer: Coming out of COVID-19 Better, Faster, Stronger
4- The post-COVID-19 pricing imperative for P&C insurers
5- Munich Re, “The next generation of pricing actuaries”, https://www.munichre.com/en/solutions/reinsurance-property-casualty/global-consulting/pricing-consulting/pricing-article-download.html
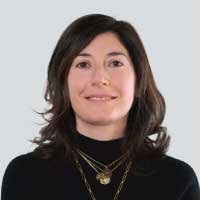
Anne-Laure Klein
Anne-Laure Klein is Chief Operating Officer at Akur8. She started her career in strategy consulting, working for 8 years at L.E.K. Consulting in Europe and Australia. She moved to the corporate world where she held various global leadership positions in strategy, digital and data transformation and digital partnerships at Carrefour and Sodexo over the course of 9 years. Anne-Laure graduated from ESSEC Business School and holds an MBA from INSEAD.
Anne-Laure Klein
Anne-Laure Klein is Chief Operating Officer at Akur8. She started her career in strategy consulting, working for 8 years at L.E.K. Consulting in Europe and Australia. She moved to the corporate world where she held various global leadership positions in strategy, digital and data transformation and digital partnerships at Carrefour and Sodexo over the course of 9 years. Anne-Laure graduated from ESSEC Business School and holds an MBA from INSEAD.